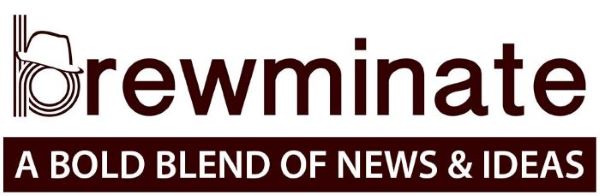
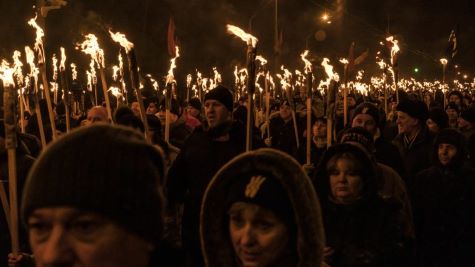
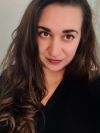
By Dr. Aleksandra Urman
Postdoctoral Researcher
Institute of Communication and Media Studies, University of Bern
Social Computing Group, University of Zurich
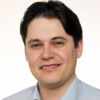
By Stefan Katz
Institute of Digital Enabling
Bern University of Applied Sciences
Abstract
The present paper contributes to the research on the activities of far-right actors on social media by examining the interconnections between far-right actors and groups on Telegram platform using network analysis. The far-right network observed on Telegram is highly decentralized, similarly to the far-right networks found on other social media platforms. The network is divided mostly along the ideological and national lines, with the communities related to 4chan imageboard and Donald Trump’s supporters being the most influential. The analysis of the network evolution shows that the start of its explosive growth coincides in time with the mass bans of the far-right actors on mainstream social media platforms. The observed patterns of network evolution suggest that the simultaneous migration of these actors to Telegram has allowed them to swiftly recreate their connections and gain prominence in the network thus casting doubt on the effectiveness of deplatforming for curbing the influence of far-right and other extremist actors.
Introduction
Far-right1 actors frequently claim that mainstream media are biased against them, misrepresent their opinions or ignore them altogether (Ellinas, 2018; Marwick & Lewis, 2017). US President Donald Trump regularly accuses mainstream media of spreading fake news (Carson & Farhall, 2019). Frauke Petry, chair of Alternative for Germany, has repeatedly criticized the media for ‘lying’ (Wiget, 2016). Leader of the Dutch Party for Freedom told the press to ‘drop dead’ (‘Drop dead suckers, Geert Wilders tells politicians and the press,’, 2015), and far-right PEGIDA movement in Germany promotes the term ‘Lügenpresse’ (‘lying press’).
Given their hostility towards mainstream press, it is not surprising that far-right actors actively use new media for mobilization, coordination and information dissemination (Mudde, 2019; Schroeder, 2018). In fact, the recent rise of far right is arguably driven by digital media (Jungherr et al., 2019). Online platforms allow far-right actors to bypass the gatekeepers of institutional political discourse, such as parties and mainstream media (Schroeder, 2018), and make it easier for them to promote extremist messages and hate speech without being investigated by the police (Tateo, 2005).
Social media have been widely used by far-right actors in the last decade (Jungherr et al., 2019). Though useful for all far-right actors, they are particularly important for smaller, and often more extreme, far-right subcultures, some of which exist exclusively online, than for the bigger well-organized groups such as radical right political parties (Mudde, 2019, p. 112). This makes digital trace data crucial to research on far-right groups. Striving to curb hate speech, major social media platforms (i.e., Facebook and Twitter) have recently begun deplatforming (permanently banning) far-right actors. As a result, far right has started migrating to other platforms, normally those that have less strict moderation of hate speech (Rogers, 2020). Studying the activities of far right on the platforms they move to is important for understanding the effects of deplatforming on these groups and their development outside mainstream social media. In this paper, we focus on Telegram – a messaging platform that has become increasingly used by far right in 2019, after a series of bans by Facebook and Twitter (Rogers, 2020).
Related Research
The analysis of the online activities of far-right groups can help uncover the discourse promoted by the far-right groups (Atton, 2006) and, in some cases, the effects of the promoted messages (Müller & Schwarz, 2018); as well as get insights about the connections between different far-right organizations and online outlets (Tateo, 2005).
One of the earliest studies that examined online activities of the far right employed network analysis to explore the interconnections between selected white supremacist websites in the US and found that this network is decentralized and consists of several distinct communities (Burris et al., 2000). Studies relying on the same methodology revealed that the networks of Russian (Zuev, 2011) and Italian (Tateo, 2005) right-wing websites are similarly decentralized, while the network of German right-wing websites is more centralized (Caiani & Wagemann, 2009). Yet another group of researchers examined the network of users who contribute to racist and hate speech blogs and found that it is decentralized and divided into several clusters (Chau & Xu, 2007).
A different stream of scholarship focused on the content of the far-right websites rather than on the interconnections between them. Scientists analyzed the content of 157 right-wing extremist websites in the US (Gerstenfeld et al., 2003); the discourse of the British National Party’s website (Atton, 2006); the narratives of an online skinhead newsgroup (Campbell, 2006); the rhetoric of restrictionist online groups in the US in the aftermath of 9/11 (Sohoni, 2016).
Though far-right actors actively use social media platforms for communication and mobilization (Ellinas, 2018), until recently only few studies had explored the activities of the far right on these platforms. One of the first such studies (O’Callaghan et al., 2013) relied on a network-analytic approach and the data about the activities of far-right Twitter users to examine transnational links between them in the form of retweets and mentions. Another one used similar method to investigate transnational connections between far-right organizations (Froio & Ganesh, 2018). Researchers also combined a network analytic approach with content analysis to examine the networks and discourses of Western European far-right groups’ Facebook pages (Klein & Muis, 2019).
Recently, a rather big body of research exploring the communication of a specific part of the far-right spectrum, namely populist radical right parties and groups, on social media has emerged. This research examined psychological traits of young people following Facebook pages of right-wing populist actors (Heiss & Matthes, 2017); compared communication styles of PEGIDA movement and German political parties (Stier et al., 2017); explored the representation of mainstream media in the messages posted by the PEGIDA Facebook groups in Germany and Austria (Haller & Holt, 2019); analyzed the relationship between anti-immigrant attitudes and selective exposure to populist radical right content on Facebook (Heiss & Matthes, 2019) and association between the anti-refugee sentiment on Facebook promoted by Alternative for Germany and increase in violent crimes against refugees (Müller & Schwarz, 2018).
The aforementioned studies have helped advance our understanding of strategies employed by the far right to gain attention on social media, the effects of their messages, and the interconnections between these groups and their audiences. Still, we suggest that there is a research gap related to the communication of and interconnections between the far-right actors on social media. First, most of the recently published papers that deal with far right on social media have focused on populist radical right parties, though social media are of lesser importance to such big and organized groups than to more marginal and extreme far-right subcultures (Mudde, 2019). Second, all of the studies referred to above have focused on what can be called mainstream social media platforms – Facebook and Twitter. However, in the recent years these platforms have started banning far-right actors (Al-Heeti, 2019; London, 2019). This, in turn, leads to the ‘migration’ of the far-right actors to other platforms. For instance, as media reports demonstrate, in 2016, after a wave of Twitter bans, far-right users started switching to social network called Gab (Wilson, 2016), and in 2019 messaging app Telegram has become increasingly popular with the far right (Owen, 2019; Rogers, 2020). In the next section, we describe this platform in greater detail and discuss why its affordances – possibilities for different user actions (Bucher & Helmond, 2018) – make it attractive to far right actors and important to examine for the scholars focusing on the communication of far right and other fringe actors.
Telegram: The Quest for Privacy
Telegram is a free cloud-based instant messaging platform created in August 2013 by privacy-keen entrepreneurs, brothers Pavel and Nikolai Durov, amid their own troubles with the authoritarian Russian state over the freedom of speech (Miller, 2015). It comes as no surprise that the platform is privacy-focused as underscored in its FAQ:
If criticizing the government is illegal in some country, Telegram won‘t be a part of such politically motivated censorship. This goes against our founders’ principles. While we do block terrorist (e.g., ISIS-related) bots and channels, we will not block anybody who peacefully expresses alternative opinions (Telegram F.A.Q., n.d.). In line with its privacy focus, Telegram supports multiple privacy-enhancing features. These include:
- Channels (public and private). Using a channel, its creator and/or administrators can send messages to an unlimited number of subscribers. Messages are signed by the channel’s name, so the sender (administrator) can remain anonymous since even subscribers do not see who created and/or administrates the channel. Channels can be public – searchable (one can find them by keywords/name searching on Telegram) and visible to all Telegram users – or private – not searchable (not returned in search results) and visible only to the users who were invited by the administrator via a direct link.
- Groups (public and private group chats). These are similar to group chats on other platforms (i.e., WhatsApp). Public groups are searchable, any user can join them or be invited by any group member. Maximum number of members in a public group is 200000. Private groups are not searchable, and new members can join only by invitation from the group administrator. Public groups are thus useful for mass coordination and discussion, and private ones for more secretive communication.
- Encryption. All the messages (excluding those from Secret chats – see below) are stored on Telegram’s servers and use client-server/server-client encryption with the encryption keys stored in multiple locations so local technical personnel cannot access messages hosted on a given server (Telegram Privacy Policy, n.d.)
- Secret chats. Messages sent through these chats use end-to-end encryption so only the sender and the recipient can access them. They are not stored on Telegram’s servers and can be accessed only from the devices on which they were sent/received. Secret chats can self-destruct after a specified period. Telegram deletes all logs about secret chats making it impossible to later infer whether secret chat users contacted each other (Telegram Privacy Policy, n.d.). According to Telegram, ‘Secret chats are meant for people who want more secrecy than the average fella’ (Telegram F.A.Q., n.d.).
- Anonymous forwarding. With this setting, messages forwarded to others no longer point to the original sender’s account (the forwarded message displays an unclickable ‘from’ form). As Telegram states, ‘This way people you chat with will have no verifiable proof you ever sent them anything.’ (Taking Back Our Right to Privacy, 2019)
- Unsend anything. A user can immediately delete any message they sent or received. This feature can be relevant, for instance, if one’s phone is (about to be) seized by law enforcement agencies and messages can be used as evidence against a user.
By offering enhanced privacy and anonymity along with opportunities to gain publicity (through channels) and coordinate and mobilize (though groups), Telegram offers a solution to the so-called terrorist’s (Rogers, 2020; Shapiro, 2013) – or online extremist’s – dilemma that concerns balancing ‘public outreach and operational security in choosing which digital tools to utilize’ (Clifford & Powell, 2019). This makes the platform attractive for users who need publicity and mobilization opportunities while preserving anonymity to avoid legal consequences and/or persecution. Such groups range from protesters and activists in authoritarian regimes, as examples of Telegram-aided mobilization in Hong Kong, Russia and Iran (Banjo, 2019; Ebel, 2019; Karasz, 2018) show, to extremist groups including Islamic State (Krona, 2020) and various far-right organizations in the West (Rogers, 2020).
There is evidence that Telegram is getting increasingly popular with the far right, especially in light of the moves by Facebook and Twitter to block their content, and the shutdown of the 8chan image-board that used to be popular among the extreme right (Owen, 2019). The reach of the far-right actors on Telegram is much lower than on Facebook or Twitter (Rogers, 2020), meaning that the platform might not be very helpful for the far right to attract new followers. However, due to the combination of Telegram’s affordances and the absence of moderation of far-right content – unlike on Facebook or Twitter – there is increased likelihood of users’ radicalization. On Telegram users can communicate in ideologically homogenous groups and distribute extremist content without fearing any legal consequences, with anonymity and ideological homogeneity increasing radicalization potential (Mudde, 2019). For these reasons, we suggest that the examination of the activities of far-right actors on Telegram is relevant and necessary. So far, Telegram has been overlooked by the scholars of far-right communication with the exception of a study by Rogers (2020) that has examined the effects of deplatforming on the audience sizes of certain extremist internet celebrities after their move from mainstream platforms to alternative sites, including Telegram. Our study aims to partially fill the existing gap by complementing the analysis of Rogers (2020) and examining the interconnections between far-right channels, their links to different (non far-right) political channels as well as the evolution of far-right network on Telegram.
Research Questions and Hypotheses
First, we scrutinize the claims that the presence of the far right on Telegram significantly increased in 2019, after mainstream social media platforms banned many prominent far right accounts (Owen, 2019). There is evidence that a handful of prominent far-right internet celebrities have moved to Telegram (Rogers, 2020). However, we intend to test whether the platform migration involved a wide circle of far-right actors. Our first hypothesis stems from this intention.
H1: Bans of far-right accounts on mainstream social media (e.g., Facebook, Twitter, Instagram) are associated with the increase in the presence of similar accounts on Telegram.
We cannot test whether there is a direct causal relationship between the bans of far right on mainstream social media and the increase in their presence on Telegram. However, we analyze whether the spikes in the growth rate of the far-right network on Telegram coincide in time with the bans of far-right actors on other platforms. If they do, we can there is an association between these events which would cast doubt on the effectiveness of deplatforming to prevent the radicalization of those exposed to the content produced by the banned users. If far-right actors ‘migrate’ from well-established platforms to more obscure ones following the bans en masse, it might become more difficult for the authorities to monitor their activities and in turn lead to the radicalization of their rhetoric – on more obscure platforms they are less constrained by the moderation of hate speech.
The second aim of the present paper is to explore the structure of the far-right network on Telegram and its evolution. The first research question we strive to answer in this respect is the following:
RQ1: Is the structure of the far-right network on Telegram similar to those of the far-right networks on other platforms?
We examine the community structure of the far-right network on Telegram and compare the findings to those about the far-right networks on Twitter (Froio & Ganesh, 2018; O’Callaghan et al., 2013) and Facebook (Klein & Muis, 2019). Based on the previous findings, we expect the Telegram network to be decentralized and divided into distinct communities. If that is the case, then the structure of far-right networks is similar across platforms and is re-created under different conditions suggesting that exogenous factors (e.g., ideological divisions within the far right) have stronger influence on shaping the network than platform affordances, and that following deplatforming, far-right actors recreate structures that existed on those they were banned from.
To better understand which factors might affect the network structure and along which lines the network is divided we answer the following question:
RQ2: What are the major communities that comprise the far-right network on Telegram?
Since the structures found in online networks tend to reflect those observed in the corresponding offline networks (Dunbar et al., 2015), we expect to observe divisions along ideological lines with communities corresponding to different far-right subcultures. In addition, we aim to examine other division lines (i.e., national or linguistic).
We also explore the following question:
RQ3: How is the far-right network connected to non-far-right communities?
Previous studies into far-right networks on social media focused solely on the far-right cores of such networks without examining how the actors and organizations comprising these networks are connected to the groups that have little to do with the far-right ideology. Our data collection methodology allows us to address this limitation. We find this research question important since it is helpful for understanding through which channels the messages from far-right actors spread to the broader public. This might be of special importance in the context of Telegram where the far-right actors do not have a broad reach yet (Rogers, 2020), and thus might seek to circulate their messages as widely as possible to attract new followers.
Finally, we look at the evolution of the far-right network and examine the following:
RQ4: How did the composition and structure of the far-right network on Telegram change overtime?
Aforementioned studies into far-right networks on social media have examined their structure disregarding the dynamics of the network evolution. They looked into the networks after summing up all the interactions that took place at different times, thus collapsing a dynamic network into a single snapshot. We suggest that including temporal dimension in the analysis allows to get more nuanced data about the network structure and see how it evolved. Our methodology allows to trace the development of the far-right network on Telegram from the very beginning, which is especially useful for understanding how far-right actors (re)create communication structures following deplatforming.
Data and Methods
Data Collection
The data was collected using Telegram’s open API and Telethon Python library (Exo, 2020) using Exponential Discriminative Snowball sampling (Baltar & Brunet, 2012; Biernacki & Waldorf, 1981; Goodman, 1961). The initial seed was selected by the authors which is appropriate to reach hidden populations with specific properties (Etikan, 2016). To collect the messages and mentions we created a Telegram bot (referred to as probe below) and chose @martinsellnerib channel as the starting seed. This is the official channel of Martin Sellner, an Austrian far-right (Identitarian) activist. We chose this specific seed since at the time when we started the data collection Sellner’s channel was the most popular (in terms of the number of subscribers) far-right channel among Telegram’s political channels according to tgstat.com website that collects and publishes the rankings of Telegram channels.
The probe started with collecting the messages for @martinsellnerib. Then we extracted mentions of other channels and/or public groups2 from the collected messages. The process is illustrated in Figure 1.
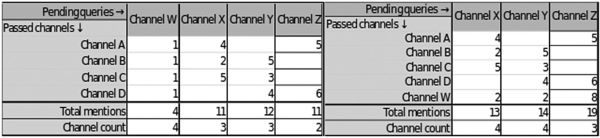
Channels A, B, C, D have already been sampled and their mentions of channels W, X, Y and Z have been analyzed. The matrix is read as ‘Channel W has been mentioned by Channel A one time.’ In this example, the next channel to be sampled is Channel W because it is mentioned by the highest number of channels at this point. After sampling Channel W, the sampled mentions are sorted again, now including Channel W, and the next channel is determined (see Figure 1). In this second iteration, the channel Y would be sampled, as both channels X and Y are mentioned by 4 channels, but Channel Y is mentioned more often by the sampled channels. This prioritization was chosen to reduce the impact of large channels that mention few channels many times and with the objective of creating a dense network.
Since the starting channel was not chosen at random, some bias was introduced in the data collection due to the nature of the sampling procedure. We elaborate on this below.
Overall, we collected the data on 53296 channels, public and private3 groups (including 1744 with full histories of their posts and 51552 that were mentioned by them, without the full histories available for the latter). The corresponding data spans over a period from September 2015 – when the first channels included in the network were created – to February 2020. Subsequently, we created a citation network using all the mentions from the 1744 channels with full histories (self-citations were excluded). Mentions include both, direct reposts from other channels/groups and mentions of other channels/group chats in message texts. The sentiment of the latter (positive/negative referral in the text) was not taken into account. The resulting network has 53296 nodes and 219314 weighted (weight equals the number of mentions; the total number of mentions (unweighted edges) is 5532098) edges.
Methods
Methodologically, this paper follows in the footsteps of the researchers who relied on network analysis to examine the interconnections between different far-right groups and accounts (Burris et al., 2000; Caiani & Wagemann, 2009; Chau & Xu, 2007; Ellinas, 2018; Froio & Ganesh, 2018; O’Callaghan et al., 2013; Tateo, 2005; Zuev, 2011). To answer the research questions 1, 2 and 3, we employed methods similar to those used by the other researchers. Specifically, we collapsed the network into one snapshot, disregarding the temporal dimension, and then applied community detection algorithm to it (Blondel et al., 2008), and looked at the descriptive network statistics (i.e., node in-degrees, out-degrees, etc). The community detection algorithm allows to separate the network into groups of nodes that are more closely connected to the nodes within a given community than to the nodes outside of it. In the present study, it means that the channels within a given community cite and are cited by the channels from the same community more than by the channels from the other communities.
To test hypothesis 1 and answer research question 4 the method mentioned above is, however, not suitable since it disregards the dynamic aspect of the network formation. Hence, to trace the evolution of the network, we employed methods that take into account temporal dimension. In order to test hypothesis 1, we first divided the network into several snapshots, each corresponding to one month. Then we checked the descriptive statistics related to network growth. Specifically, we looked at the rates of edge formation and node creation corresponding to each month. Then we checked whether there are spikes in the network growth and, if so, whether they coincide in time with the massive bans of far-right accounts on mainstream social media.
Finally, to answer research question 4, we repeated the analysis done to answer research questions 1, 2, 3, for the smaller network snapshots each corresponding to one month which we generated for testing H1. Then, using the insights about the spikes in the network growth obtained while testing H1, we qualitatively analyzed the evolution of the far-right network during the periods of its most active growth and described the changes that took place in the network structure during this time.
Results
RQ1: the far-right network on Telegram is decentralized
Community detection algorithm (Blondel et al., 2008) applied to the final snapshot of the far-right network on Telegram4 divided the network into 28 communities with a modularity score of 0.725. The modularity score suggests that the network has a very distinct community structure – the metric varies between 0 and 1 with higher scores indicating stronger structure (Newman & Girvan, 2004). In practice, scores above 0.7 are rarely observed (González-Bailón & Wang, 2016) meaning that the community structure is particularly strong in this case. Hence, the observed network is highly decentralized and divided into multiple groups, similarly to the far-right networks on other platforms (Froio & Ganesh, 2018; Klein & Muis, 2019; O’Callaghan et al., 2013).
RQ2: the divisions in the network
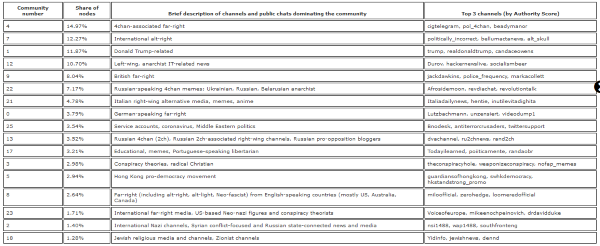
The distribution of communities found in the network is presented in Table 1. The ‘Community number’ column can be used for reference to match the community description to its position in the network (Figure 2) using the provided color legend. The representation of the network in Figure 2 was rendered using a force directed ForceAtlas2 algorithm (Jacomy et al., 2014) implemented in Gephi. In Table 1 we present how big each community is relative to the network which is measured by the share of nodes it encompasses. We also provide a brief description of each community which we obtained after qualitatively examining the top-20 channels ordered by Authority score (Kleinberg, 1998) in a given community, and list the top-3 channels’ names for reference.
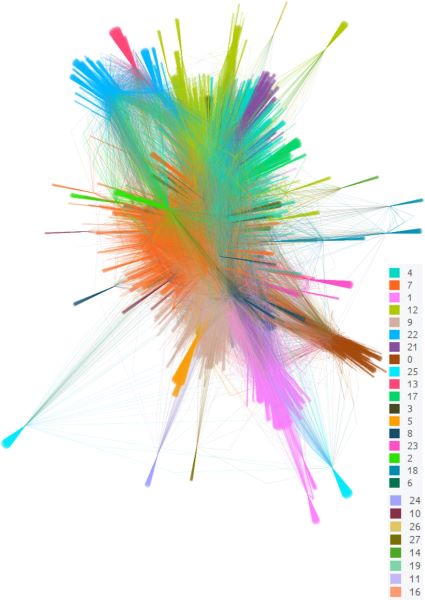
As seen in Table 1, the network has several far-right communities. The division occurs primarily along two lines: national and ideological. For instance, there are distinct British [community number 9], Italian [21], German-speaking [0], and Russian-speaking [13] communities. In the case of Italian, Russian- and German-speaking communities it is impossible to say whether their separation from other groups is driven primarily by the linguistic differences or the differences in the national contexts. However, British community is distinguished due to its specific national context, distinct from those of other English-speaking ones.
The ideological division is persistent, though not entirely clear-cut. The far right in the offline world is extremely heterogenous (Mudde, 2019) which is reflected in the observed Telegram network. The biggest communities in the network are those of alt-right [7] and various far-right channels, mostly alt-right or neo-nazi ones, associated with the 4chan image-board [4]. More mainstream and organized far-right actors, i.e., political parties, – are not present in our network since they are not represented on Telegram. Major far-right political parties do not have official Telegram channels and unofficial, if exist, are not popular – for example, as of June 2020 Alternative for Germany’s unofficial channel (@AfDInfo) had only 21 subscribers. The only mainstream political actor present in the network is Donald Trump. In fact, channels devoted to Trump comprise the third-biggest community in the network [1]. They include channels that post Trump’s statements or tweets (it is unclear if any of these are officially affiliated with him or the White House) and personal channels of his active supporters such as Candace Owens. There are separate communities that include radical Christian and conspiracy-spreading channels/groups [3], alternative right-wing media [6], as well as globally focused [2] and US-focused [23] neo-nazi channels and chats. Besides, there is an ideologically heterogenous community that includes channels of different far-right figures from English-speaking countries with the exception of Britain [8].
RQ3: how far-right communities are connected to non-far-right ones
There are several communities not related to far-right ideology per se. The biggest among them are the international left-wing and anarchist ones [22, 12] and that of Hong Kong’s pro-democracy movement [5]. To examine how the connections across different communities are facilitated, we analyzed the brokerage (Gould & Fernandez, 1989) in the network and identified major gatekeepers (channels/groups connecting nodes from other communities to the nodes in their community) and representatives (the opposite of gatekeepers).
The most important representative nodes from the far-right communities – channels/groups facilitating the spillover of messages from one community to the others – are channels related to 4chan (i.e., @randomanonch) and/or posting predominantly racist memes (i.e., @breadpilled) and far-right-related group chats (i.e., @clownworldgroup; @villiesullivanchat; @patriotictalk). In turn, the most important gatekeepers in non-far-right communities – bringing the messages from the far-right channels/groups to non-far-right communities – are also group chats: @leftism and @metaanarchomemes (groups where left-wing users among others mock right-wing channels) [12], and group chat @swhkdemocracy links the community of British far-right [9] to that related to Hong Kong’s pro-democracy movement [5]. Group chats also drive the spillover from the left-wing community [12] to the far-right ones as the major representative nodes of this community besides @leftism and @metaanarchomemes include @discordiansociety and @socialismbar.
Notably, there are no large apolitical communities. Those that are not dominated by an ideological focus either deal with certain political issues [25] or include a considerable share of political channels among the top-20 channels in the community [17].
H1 and RQ4: the evolution of the far-right network
To answer the research question about the evolution of the far-right network we looked at the dynamics of its formation. First, we looked at the speed of network development overtime to find the tipping points in network formation and test Hypothesis 1. We divided the network into 54 snapshots, each corresponding to one month, and calculated the number of new source nodes (channels/groups for which we have full histories downloaded) and edges (connections between channels in the form of citations or mentions) were created each month (Figures 3 and 4).
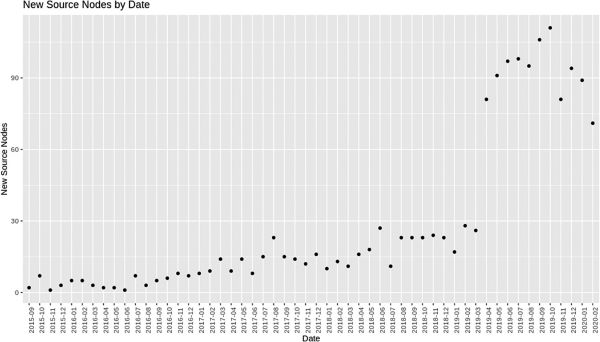
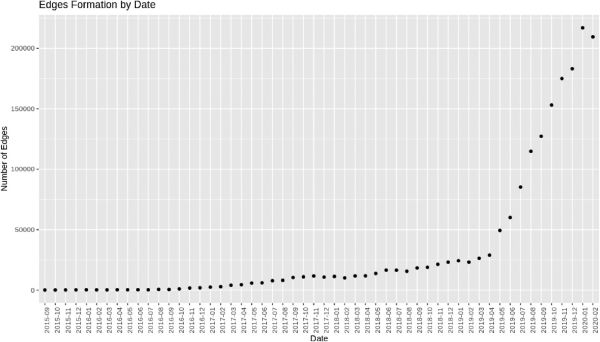
As shown in Figure 3, the most drastic increase in the number of new nodes occurred in April 2019, while the number of created edges dramatically increased in May 2019 (Figure 4) and since then has been growing more or less exponentially.
Thus, the growth of the observed network sped up significantly in April-May 2019. Importantly, the majority of active channels that joined in April 2019 are related to the British far-right actors that were banned from Facebook at that time or a bit earlier – in February 2019. This means that the rapid growth observed in April-May 2019 is attributed to the growth of the far-right part of the network.
In April and May 2019 major bans of far-right accounts took place on Facebook and Instagram (Hern, 2019). We suggest that the rapid growth of the far-right network on Telegram is connected to these bans and was led by the migration of British far-right groups to Telegram in April 2019 after they were banned from Facebook. This partially supports Hypothesis 1. However, we cannot say that it is fully supported: bans of far-right figures had also happened before spring 2019 on Facebook, Instagram and Twitter (Stephen, 2019) and for those periods we do not observe a significant influx of far-right channels on Telegram. We outline possible reasons for this in the Discussion.
Since the channels that form the core of the far-right part of the network mostly joined in spring 2019 or later, when addressing research question 4, we focused on the network evolution starting from March 2019. We created separate network snapshots corresponding to each month from March 2019 to February 2020. For the sake of space limit, we do not provide the snapshots of the networks here but present the overview of the top communities in each monthly snapshot in Table 2.5
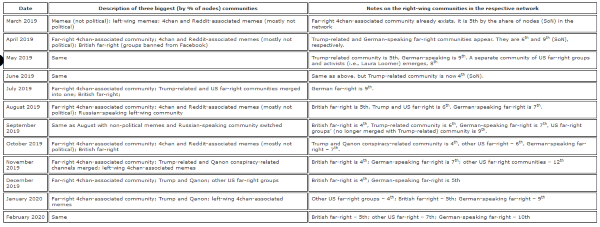
In April 2019 the far-right channels/group chats associated with 4chan became dominant in the network and have maintained this status throughout the observation period. At the same time, distinct British and German-speaking far-right communities emerged, as well as a community related to Donald Trump. In May 2019 another distinct community of the US far-right appeared coinciding in time with bans of far-right actors on Facebook and Instagram. Over the next months the aforementioned communities have been switching positions in the network, but all three aforementioned English-speaking far-right communities remained dominant, with Donald Trump and Qanon conspiracy-related channels/groups being particularly large.
Sampling Limitations
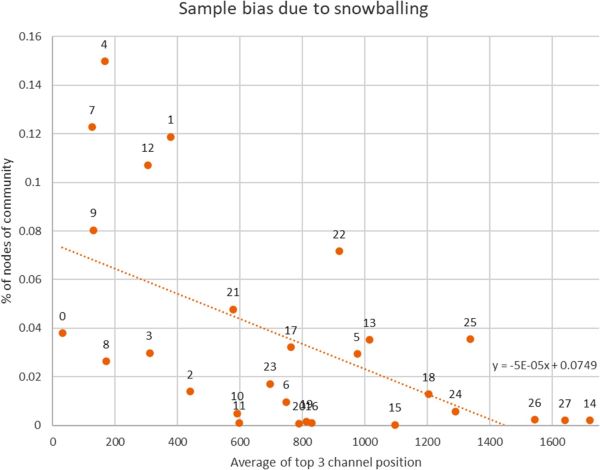
Snowball sampling has its limitations. Given that the sampled channels determine the next channel to be sampled, mentions from early channels have a higher impact on the path of the probe than later channels. The second channel after @martinsellnerib (@identitaereoesterreich) is determined from 575 mentions, whereas the 21st channel (@beimhermann) is already determined from 9206 mentions. The probe therefore prioritizes channels early in the data collection that it would not have sampled later. This increases the number of nodes of early sampled communities relative to those sampled later. Figure 5 illustrates this bias. The Figure shows the average sampling position of the top three sampled channels by authority of each community on the x-axis and the share of nodes of this community on the y-axis. The probe collected (in relative terms) more nodes from German-speaking [0] and English far-right [7] communities. Smaller early communities [0,8,3] might not have been sampled as distinct communities if a different starting seed had been chosen.
Discussion
Our analysis shows that the structure of the far-right network on Telegram is similar to those observed on mainstream social media platforms (i.e., Froio & Ganesh, 2018; Klein & Muis, 2019; O’Callaghan et al., 2013) – the observed network is decentralized and divided into several distinct communities. The divisions mainly occur along the ideological and national lines. This finding suggests that far-right actors tend to recreate similar structures on different platforms regardless of the platform affordances.
The biggest community in the far-right core of the network is the one associated with the alt-right groups on the 4chan platform. One explanation could be that this community is more active and organized than others since alt-right exists predominantly in the online sphere (Mudde, 2019). The structure of the network also shows that 4chan-related channels/group chats facilitate the connections between the far-right core of the network and the non-far-right communities. 4chan-related political communities we observe on Telegram are extremist regardless of their position on the left-right spectrum. We believe that research on 4chan itself is necessary to find out if the political communities on that platform tend to be extreme in general or what we observe on Telegram has more to do with the selection bias than with 4chan itself. Still, our analysis shows that on Telegram extreme right-wing and left-wing communities are quite large (see Table 1). Hence, we suggest that research into the corresponding groups on 4chan could trace if and how radicalization takes place among the users of the image-board as well as examine the spillover to other platforms.
We also find that Donald Trump-related channels exert significant influence in the far-right core of the network. Though we do not know whether any of them are officially associated with Trump or the White House, their prominence in the network, along with the absence of any other mainstream far-right political parties, lends additional evidence to the argument that Trump and his presidency have aided normalizing the far-right rhetoric (Mudde, 2019).
Our analysis confirms that in 2019 the presence of Western far-right on Telegram has significantly increased. Previous research (Rogers, 2020) has shown that individual far-right actors have joined Telegram in this period, while our study demonstrates that this migration was in fact rather massive. It started when a large number of far-right channels joined the platform in April 2019, following waves of bans on Facebook. Importantly, the structure of the far-right core of the analyzed network did not gradually evolve but remained similar since April 2019. Besides, far-right actors became dominant in the observed network almost immediately as they joined the platform.
We suggest that the fact that many far-right actors ‘migrated’ to Telegram simultaneously has allowed them to swiftly establish the connections with each other, thus recreating the structures that existed on the platforms from which they were banned, and quickly establishing their dominance. There is anecdotal evidence that similar phenomena were observed on Gab platform in 2016 when far-right joined it after a series of Twitter bans (Wilson, 2016). For instance, the man accused of opening fire on a synagogue in Pennsylvania killing 11 people in 2018 was an active user of Gab (Hern, 2018).
After transfers to less-regulated platforms far-right actors lose huge shares of their audiences (Rogers, 2020) but the bans also lead to the decrease in transparency about their activities. Their audiences thus get smaller but at the same time can become more radicalized. Further, we observe that the size of the audiences of far-right channels on Telegram increases overtime (see Figure 6). The numbers are low, if compared to those far-right celebrities used to enjoy on mainstream platforms. Still, there is an upward trend in the viewing numbers since August 2019 – the month when 8chan image-board went offline suggesting that former 8chan users might have flocked to Telegram and fueled the viewing numbers of far-right channels there.
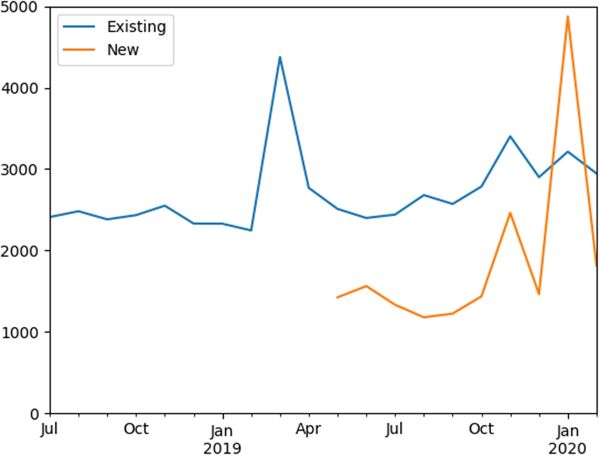
We propose that outright bans of extremist users and groups might not be the best way to curb their influence and prevent users’ radicalization. Bans that are administered gradually and applied to few actors at a time might be a better solution. They would hamper the coordination abilities of the banned users and groups, making it harder for them to recreate the structures that existed on the older platforms and instantly become dominant on the new ones. Furthermore, alternative measures could be used to limit their reach: i.e., new posts from the users who had published extremist content before might be downgraded by the social media algorithms so fewer people see them; temporary bans on the sharing and/or commenting on the posts written by such users can be introduced. We argue that more research into the effects of bans and their alternatives is necessary to establish which measures work best to not just limit the reach of extremist actors in short-term but curb the radicalization of their audiences in the long run.
Appendix
Notes
- Following Mudde (2007, 2019), in this paper we use “far right” as umbrella term that includes different subgroups such as extreme right and radical right.
- All the data collected is publicly available to any Telegram user, and, for ethical reasons, in the course of the analysis we relied only on aggregated data without attributing any messages to individual users. Private channels when mentioned are represented with hashes only so one cannot access them directly.
- No content collected for private groups/channels.
- See supplementary material for the corresponding data.
- See supplementary material for the corresponding data.
References
- Al-Heeti, A. (2019, February 26). Twitter, Facebook ban far-right figures for fake accounts, hate speech. CNET. https://www.cnet.com/news/twitter-facebook-ban-far-right-figures-for-fake-accounts-hate-speech/[Google Scholar]
- Atton, C. (2006). Far-right media on the internet: Culture, discourse and power. New Media & Society , 8 (4), 573–587. https://doi.org/10.1177/1461444806065653[Crossref], [Web of Science ®], [Google Scholar]
- Baltar, F. , & Brunet, I. (2012). Social research 2.0: Virtual snowball sampling method using Facebook. Internet Research , 22 (1), 57–74. https://doi.org/10.1108/10662241211199960[Crossref], [Web of Science ®], [Google Scholar]
- Banjo, S. (2019, August 15). Hong Kong protests drive surge in Telegram Chat App. Bloomberg.Com. https://www.bloomberg.com/news/articles/2019-08-15/hong-kong-protests-drive-surge-in-popular-telegram-chat-app[Google Scholar]
- Biernacki, P. , & Waldorf, D. (1981). Snowball sampling: Problems and techniques of chain referral sampling. Sociological Methods & Research , 10 (2), 141–163. https://doi.org/10.1177/004912418101000205[Crossref], [Web of Science ®], [Google Scholar]
- Blondel, V. D. , Guillaume, J.-L. , Lambiotte, R. , & Lefebvre, E. (2008). Fast unfolding of communities in large networks. Journal of Statistical Mechanics: Theory and Experiment , 2008 (10), https://doi.org/10.1088/1742-5468/2008/10/P10008[Crossref], [Google Scholar]
- Bucher, T. , & Helmond, A. (2018). The affordances of social media platforms . [Google Scholar]
- Burris, V. , Smith, E. , & Strahm, A. (2000). White supremacist networks on the internet. Sociological Focus , 33 (2), 215–235. https://doi.org/10.1080/00380237.2000.10571166[Taylor & Francis Online], [Google Scholar]
- Caiani, M. , & Wagemann, C. (2009). Online networks of the Italian and German extreme right. Information, Communication & Society , 12 (1), 66–109. https://doi.org/10.1080/13691180802158482[Taylor & Francis Online], [Web of Science ®], [Google Scholar]
- Campbell, A. (2006). The search for authenticity: An exploration of an online skinhead newsgroup. New Media & Society , 8 (2), 269–294. https://doi.org/10.1177/1461444806059875[Crossref], [Web of Science ®], [Google Scholar]
- Carson, A. , & Farhall, K. (2019, October 1). The real news on “fake news”: Politicians use it to discredit media, and journalists need to fight back. The Conversation. http://theconversation.com/the-real-news-on-fake-news-politicians-use-it-to-discredit-media-and-journalists-need-to-fight-back-123907[Google Scholar]
- Chau, M. , & Xu, J. (2007). Mining communities and their relationships in blogs: A study of online hate groups. International Journal of Human-Computer Studies , 65 (1), 57–70. https://doi.org/10.1016/j.ijhcs.2006.08.009[Crossref], [Web of Science ®], [Google Scholar]
- Clifford, B. , & Powell, H. C. (2019, June 6). De-platforming and the online extremist’s dilemma. Lawfare. https://www.lawfareblog.com/de-platforming-and-online-extremists-dilemma[Google Scholar]
- Drop dead suckers, Geert Wilders tells politicians and the press . (2015, December 21). DutchNews.Nl. https://www.dutchnews.nl/news/2015/12/drop-dead-suckers-geert-wilders-tells-politicians-and-the-press/[Google Scholar]
- Dunbar, R. I. M. , Arnaboldi, V. , Conti, M. , & Passarella, A. (2015). The structure of online social networks mirrors those in the offline world. Social Networks , 43 , 39–47. https://doi.org/10.1016/j.socnet.2015.04.005[Crossref], [Web of Science ®], [Google Scholar]
- Ebel, F. (2019, September 15). Outlawed app Telegram emerges as key tool for Russian protesters. The Denver Post. https://www.denverpost.com/2019/09/15/russia-protest-telegram-app/[Google Scholar]
- Ellinas, A. A. (2018). Media and the radical right ( J.Rydgren , Ed.; Vol. 1). Oxford University Press. https://doi.org/10.1093/oxfordhb/9780190274559.013.14[Google Scholar]
- Etikan, I. (2016). Comparision of snowball sampling and sequential sampling technique. Biometrics & Biostatistics International Journal , 3 (1), https://doi.org/10.15406/bbij.2016.03.00055[Crossref], [PubMed], [Google Scholar]
- Exo, L. (2020). Telethon: Full-featured Telegram client library for Python 3 (1.11.2) [Python]. https://github.com/LonamiWebs/Telethon[Google Scholar] Froio, C. , & Ganesh, B. (2018). The transnationalisation of far right discourse on Twitter. European Societies , 1–27. https://doi.org/10.1080/14616696.2018.1494295[Google Scholar]
- Gerstenfeld, P. B. , Grant, D. R. , & Chiang, C.-P. (2003). Hate online: A content analysis of extremist internet sites. Analyses of Social Issues and Public Policy (ASAP) , 3 (1), 29–44. https://doi.org/10.1111/j.1530-2415.2003.00013.x[Crossref], [Google Scholar]
- González-Bailón, S. , & Wang, N. (2016). Networked discontent: The anatomy of protest campaigns in social media. Social Networks , 44 , 95–104. https://doi.org/10.1016/j.socnet.2015.07.003[Crossref], [Web of Science ®], [Google Scholar]
- Goodman, L. A. (1961). Snowball sampling. Annals of Mathematical Statistics , 32 (1), 148–170. https://doi.org/10.1214/aoms/1177705148[Crossref], [Google Scholar]
- Gould, RV , & Fernandez, RM. (1989). Structures of Mediation: A Formal Approach to Brokerage in Transaction Networks. Sociological Methodology , 19 , 89–126. https://doi.org/10.2307/270949[Crossref], [Google Scholar]
- Haller, A. , & Holt, K. (2019). Paradoxical populism: How PEGIDA relates to mainstream and alternative media. Information, Communication & Society , 22 (12), 1665–1680. https://doi.org/10.1080/1369118X.2018.1449882[Taylor & Francis Online], [Web of Science ®], [Google Scholar]
- Heiss, R. , & Matthes, J. (2017). Who ‘likes’ populists? Characteristics of adolescents following right-wing populist actors on Facebook. Information, Communication & Society , 20 (9), 1408–1424. https://doi.org/10.1080/1369118X.2017.1328524[Taylor & Francis Online], [Web of Science ®], [Google Scholar]
- Heiss, R. , & Matthes, J. (2019). Stuck in a nativist spiral: Content, selection, and effects of right-wing populists’. Communication on Facebook. Political Communication , 1–26. https://doi.org/10.1080/10584609.2019.1661890[Google Scholar]
- Hern, A. (2018, October 29). Gab forced offline following anti-semitic posts by alleged Pittsburgh shooter. The Guardian. https://www.theguardian.com/technology/2018/oct/29/gab-forced-offline-following-anti-semitic-posts-by-pittsburgh-shooter[Google Scholar]
- Hern, A. (2019, April 18). Facebook bans far-right groups including BNP, EDL and Britain First. The Guardian. https://www.theguardian.com/technology/2019/apr/18/facebook-bans-far-right-groups-including-bnp-edl-and-britain-first[Google Scholar]
- Jacomy, M. , Venturini, T. , Heymann, S. , & Bastian, M. (2014). Forceatlas2, a continuous graph layout algorithm for handy network visualization designed for the Gephi software. PLOS ONE , 9 (6), e98679. https://doi.org/10.1371/journal.pone.0098679[Crossref], [PubMed], [Web of Science ®], [Google Scholar]
- Jungherr, A. , Schroeder, R. , & Stier, S. (2019). Digital media and the surge of political outsiders: Explaining the success of political challengers in the United States. Germany, and China. Social Media + Society , 5 (3), https://doi.org/10.1177/2056305119875439[Google Scholar]
- Karasz, P. (2018, May 2). What Is Telegram, and why are Iran and Russia trying to ban it? The New York Times. https://www.nytimes.com/2018/05/02/world/europe/telegram-iran-russia.html[Google Scholar]
- Klein, O. , & Muis, J. (2019). Online discontent: Comparing Western European far-right groups on Facebook. European Societies , 21 (4), 540–562. https://doi.org/10.1080/14616696.2018.1494293[Taylor & Francis Online], [Web of Science ®], [Google Scholar]
- Kleinberg, J. M. (1998). Authoritative sources in a hyperlinked environment. Proceedings of the Ninth Annual ACM-SIAM Symposium on Discrete Algorithms, 668–677. [Google Scholar]
- Krona, M. (2020). Mediating Islamic state collaborative media practices and interconnected digital strategies of Islamic state (IS) and Pro-IS supporter networks on Telegram. International Journal of Communication , 14 (0), 23. https://ijoc.org/index.php/ijoc/article/view/9861. [Google Scholar]
- London, K. P. J. W. (2019, May 2). Facebook bans Alex Jones, Milo Yiannopoulos and other far-right figures. The Guardian. https://www.theguardian.com/technology/2019/may/02/facebook-ban-alex-jones-milo-yiannopoulos[Google Scholar]
- Marwick, A. , & Lewis, R. (2017). Media manipulation and disinformation online. 106. [Google Scholar] Miller, C. (2015, May 18). “Russia’s Mark Zuckerberg” takes on the Kremlin, comes to New York. Mashable. https://mashable.com/2015/05/18/russias-mark-zuckerberg-pavel-durov/[Google Scholar]
- Mudde, C. (2007). Populist radical right parties in Europe . Cambridge University Press. https://doi.org/10.1017/CBO9780511492037[Crossref], [Google Scholar]
- Mudde, C. (2019). The far right today . http://public.eblib.com/choice/PublicFullRecord.aspx?p=5967791 [Google Scholar]
- Müller, K. , & Schwarz, C. (2018). Fanning the flames of hate: Social media and hate crime [CAGE Online Working Paper Series]. Competitive Advantage in the Global Economy (CAGE). https://econpapers.repec.org/paper/cgewacage/373.htm[Google Scholar]
- Newman, M. E. J. , & Girvan, M. (2004). Finding and evaluating community structure in networks. Physical Review E , 69 (2), https://doi.org/10.1103/PhysRevE.69.026113[Crossref], [Web of Science ®], [Google Scholar]
- O’Callaghan, D. , Greene, D. , Conway, M. , Carthy, J. , & Cunningham, P. (2013). An analysis of interactions within and between extreme right communities in social media. In M.Atzmueller, A.Chin, D.Helic, & A.Hotho (Eds.), Ubiquitous social media analysis (pp. 88–107). Springer. [Crossref], [Google Scholar]
- Owen, T. (2019, October 7). How Telegram became white nationalists’ go-to messaging platform. Vice. https://www.vice.com/en_uk/article/59nk3a/how-telegram-became-white-nationalists-go-to-messaging-platform[Google Scholar]
- Rogers, R. (2020). Deplatforming: Following extreme internet celebrities to Telegram and alternative social media. European Journal of Communication , https://doi.org/10.1177/0267323120922066[Crossref], [Web of Science ®], [Google Scholar]
- Schroeder, R. (2018). Rethinking digital media and political change. Convergence , 24 (2), 168–183. https://doi.org/10.1177/1354856516660666[Crossref], [Google Scholar]
- Shapiro, J. N. (2013). The terrorist’s dilemma: Managing violent covert organizations . Princeton University Press. [Crossref], [Google Scholar]
- Sohoni, D. (2016). The ‘immigrant problem’: Modern-day nativism on the web. Current Sociology . https://doi.org/10.1177/0011392106068453[Crossref], [Google Scholar]
- Stephen, B. (2019, February 22). The provocateur who went out into the cold. The Verge. https://www.theverge.com/2019/2/22/18236819/laura-loomer-twitter-protest-ban-conservative-censorship[Google Scholar]
- Stier, S. , Posch, L. , Bleier, A. , & Strohmaier, M. (2017). When populists become popular: Comparing Facebook use by the right-wing movement pegida and German political parties. Information, Communication & Society , 20 (9), 1365–1388. https://doi.org/10.1080/1369118X.2017.1328519[Taylor & Francis Online], [Web of Science ®], [Google Scholar]
- Taking Back Our Right to Privacy . (2019, March 24). Telegram. https://telegram.org/blog/unsend-privacy-emoji[Google Scholar] Tateo, L. (2005). The Italian extreme right on-line network: An exploratory study using an integrated social network analysis and content analysis approach. Journal of Computer-Mediated Communication , 10 (2), https://doi.org/10.1111/j.1083-6101.2005.tb00247.x[Crossref], [Web of Science ®], [Google Scholar]
- Telegram F.A.Q. (n.d.). Telegram. Retrieved November 1, 2019, from https://telegram.org/faq[Google Scholar] Telegram Privacy Policy. (n.d.). Telegram. Retrieved June 15, 2020, from https://telegram.org/privacy[Google Scholar]
- Wiget, Y. (2016, June 16). Journalisten entlarven Frauke Petry als «Pinocchio». Tages-Anzeiger. https://www.tagesanzeiger.ch/ausland/europa/journalisten-entlarven-frauke-petry-als-pinocchio/story/27894613[Crossref], [Google Scholar]
- Wilson, J. (2016, November 17). Gab: Alt-right’s social media alternative attracts users banned from Twitter. The Guardian. https://www.theguardian.com/media/2016/nov/17/gab-alt-right-social-media-twitter[Crossref], [Google Scholar]
- Zuev, D. (2011). The Russian ultranationalist movement on the internet: Actors, communities, and organization of joint actions. Post-Soviet Affairs , 27 (2), 121–157. https://doi.org/10.2747/1060-586X.27.2.121[Taylor & Francis Online], [Web of Science ®], [Google Scholar]
Originally published by Information, Communication & Society (04.20.2020), republished by Taylor Francis Online under an Open Access license.